How Recent Data Shows Location-Based Car Insurance Quotes Can Vary by 287% Within the Same City
How Recent Data Shows Location-Based Car Insurance Quotes Can Vary by 287% Within the Same City - Detroit's East Side Reports 287% Higher Rates Than Downtown Districts
Within the city of Detroit, a dramatic disparity in car insurance costs has emerged, with the East Side experiencing premiums 287% higher than those in downtown areas. This substantial difference underscores how location plays a key role in determining insurance rates. It appears that factors such as crime rates and the overall perception of neighborhood safety significantly influence these premiums. Consequently, individuals residing on the East Side face a considerably greater financial burden for car insurance compared to those in other parts of Detroit, potentially creating an uneven playing field in terms of accessibility to affordable coverage.
Insurance providers often rely on neighborhood demographics and crime statistics when calculating rates, which can lead to wide variations in pricing across the city. This practice raises concerns about equity and fairness, as residents in certain areas may find themselves disproportionately impacted by higher insurance costs simply due to their location. The observed disparity highlights a need for a deeper exploration into the ways insurance pricing practices can affect residents differently based on where they live.
Examining the data reveals a stark 287% difference in car insurance rates between Detroit's East Side and its downtown core. This disparity hints at broader societal issues that often correlate with crime rates, population density, and vehicle theft – factors that can fluctuate drastically within a city's boundaries. It appears that insurance companies heavily rely on data analytics, particularly historical claims data, when calculating premiums. Areas with a history of frequent accidents or claims tend to experience higher insurance costs, which leads to questions about the equity of such assessments for residents in those communities.
Looking at the situation from a planning perspective, areas with lower levels of economic investment often face increased risk perceptions from insurers, leading to higher premiums. This seems to suggest that the lack of urban development contributes to a higher insurance burden in some neighborhoods, like the East Side. It's also interesting to consider that demographics are a key factor in insurance pricing. A concentration of uninsured or underinsured drivers in certain neighborhoods can drive up the cost for everyone, reflecting the insurer's assessment of the overall risk in that area.
Further, the availability of public transportation influences insurance costs. Locations with good public transportation systems may experience lower vehicle ownership rates, thus reducing the number of accidents and claims. This ultimately benefits residents living in these areas by lowering insurance premiums. However, it appears that some insurance providers are employing geo-targeting strategies, essentially assigning premiums based on highly localized data that might not fully represent the risk level of individual drivers.
The substantial rate difference raises a concern about how insurance companies define risk. It suggests that location, rather than solely driving behavior, significantly impacts consumer risk profiles – an aspect that some view as inherently unfair. Moreover, ongoing changes in a neighborhood can influence insurance costs. If an area experiences gentrification, for example, it could potentially see insurance rates decrease as economic improvements often lead to reduced risk and better living conditions.
Furthermore, we see that car theft rates vary substantially between Detroit's different districts. The higher rate of auto thefts in areas like the East Side is a factor influencing insurance costs. Firms factor this increased risk into their premiums to account for potentially higher payouts. In conclusion, the reliance on location-based pricing reveals underlying issues in the insurance industry. Questions arise about whether these models perpetuate socioeconomic inequality by increasing the financial burden on residents in already struggling communities. It prompts us to consider how location-based pricing can be designed in a way that is more equitable while still accounting for relevant risk factors.
How Recent Data Shows Location-Based Car Insurance Quotes Can Vary by 287% Within the Same City - Car Theft Statistics Drive ZIP Code Price Gaps in Brooklyn Neighborhoods
Car theft rates in Brooklyn have surged, leading to a noticeable difference in car insurance premiums across different neighborhoods. Insurers are using these statistics, which show a substantial rise in car thefts in recent years, to determine insurance rates. ZIP codes with higher instances of car theft see a corresponding increase in insurance costs, resulting in a wide gap in premiums within the borough. This practice raises concerns about equity, as residents in neighborhoods with higher crime rates might be forced to pay more for insurance simply due to their location. It seems that these location-based pricing models might unintentionally contribute to existing socioeconomic disparities, potentially making car insurance unaffordable for some residents. The trend of increasing car thefts, coupled with the reliance on crime statistics to determine insurance premiums, suggests a worrying development that could further exacerbate challenges for residents in certain Brooklyn neighborhoods.
In Brooklyn, the surge in car thefts, which has mirrored a wider trend across NYC and the US, is creating a ripple effect on neighborhood dynamics. The number of car thefts reported in specific ZIP codes significantly varies, with some areas experiencing rates as high as 10 out of every 1,000 vehicles. This stark contrast highlights a connection between car theft and the cost of car insurance. For instance, insurance quotes can fluctuate significantly based on a neighborhood's car theft history, leading to notable price differences across the borough.
Interestingly, how well local police are doing can be linked to the theft rates. Areas with robust community policing and active efforts to combat car theft frequently see lower incidents, influencing lower insurance premiums. This relationship suggests that crime prevention measures can have an impact on auto insurance costs. However, it's important to note that not all cars are targeted equally. Popular and easier-to-steal vehicles can drive up insurance costs even within the same ZIP code. This implies that insurers are not only looking at general neighborhood crime but also specific vehicle features when calculating risk.
The demographics of a neighborhood can also play a role. Areas with a higher concentration of younger residents, for example, often experience more car theft, which can subsequently impact how insurance providers set rates for those areas. And it's not simply a matter of history; even the recovery of a stolen vehicle in a previously high-theft area can lead to temporary dips in premiums, suggesting that insurance companies are somewhat reactive to recent events. But this dip is often short-lived, as theft patterns usually resume, highlighting the ongoing need for proactive crime prevention.
Furthermore, socioeconomic factors can worsen this issue. Areas with lower socioeconomic status often face the double-whammy of high theft rates and elevated insurance costs, creating a difficult cycle. Fortunately, it's not all doom and gloom. Planning initiatives that address urban design can have a real impact. Improving lighting and fostering a greater sense of community in a neighborhood can reduce car theft rates, which can then translate to more stable and potentially even lower insurance premiums in the long run.
Factors such as proximity to public transportation hubs also seem to influence car theft statistics. It's hypothesized that leaving cars unattended for extended periods near these hubs can make them more vulnerable, suggesting that even urban infrastructure can affect risk in unexpected ways. And even when car thefts are generally down, specific times of the year can trigger a surge. For instance, car thefts tend to increase in the summer months, often resulting in temporary increases in premiums for affected neighborhoods. This demonstrates the continuous dynamic relationship between crime trends, weather patterns, and human behavior within the city environment. The relationship between car theft and insurance premiums in Brooklyn reveals a complex interplay of factors and offers another lens through which to explore urban dynamics.
How Recent Data Shows Location-Based Car Insurance Quotes Can Vary by 287% Within the Same City - Traffic Density Maps Show Why Manhattan Rates Jump Block by Block
Traffic density maps illustrate how car insurance rates in Manhattan can fluctuate dramatically from block to block. The concentration of vehicles, coupled with pedestrian activity and the overall flow of traffic, appear to play a key role in how insurers calculate risk. Areas with heavy congestion, often those with high vehicle counts and frequent pedestrian traffic, might experience higher insurance premiums compared to less congested areas. This localized approach to pricing highlights the debate regarding fairness in the insurance industry, especially as urban congestion continues to rise and new initiatives like congestion pricing are proposed. Concerns arise about whether the reliance on neighborhood-specific data accurately and fairly reflects risk, and whether those who reside in densely populated areas bear a disproportionate financial burden. As city officials explore solutions to mitigate congestion and promote equitable urban environments, understanding the impact on insurance pricing becomes increasingly vital, necessitating careful consideration of how urban design and traffic management might influence insurance costs in the years to come.
Examining the data, it's evident that car insurance rates in Manhattan can vary significantly, even on a block-by-block basis. This granular level of pricing is likely a reflection of how insurance companies are trying to capture highly localized risk factors. Reports show that prices can differ by a remarkable 287% within the city, suggesting that traditional district-wide averages don't always capture the nuances of individual block characteristics.
One significant factor contributing to this variability appears to be the density of traffic. Areas with persistently high traffic congestion—and the associated risk of accidents—tend to have higher insurance premiums. It seems that insurers are recognizing the direct correlation between the movement of people and vehicles and the potential for accidents, especially in areas with heavy pedestrian and vehicle interactions.
Car thefts, a recurring issue in many cities, also contribute significantly to these varying premiums. Specific blocks are identified as car theft hotspots where thefts occur with higher frequency than elsewhere in the city. It's not surprising then that insurance companies take this into account when calculating risk. It's interesting to see how this kind of highly localized crime data shapes risk assessments.
Street usage patterns also play a role. Blocks with high concentrations of delivery vehicles, cyclists, and pedestrians can experience different accident rates. Insurance companies seem to be increasingly sophisticated in their analysis of such street-level activity. In turn, they likely adjust premiums in areas with frequent vehicle and pedestrian interactions.
Another critical element seems to be the historical accident data associated with a given block. The analysis of past events—where and how often accidents occur—shapes an insurer's perception of risk, leading to varying premiums depending on accident history. This highlights the extent to which past accidents influence future pricing, revealing a degree of predictive modeling in the insurance industry.
The demographics of a block can be influential as well. Specifically, areas with vibrant nightlife and a younger population tend to experience higher insurance costs due to increased vehicular accidents. This suggests that demographic features are linked to patterns of driving behavior, and those patterns are factored into pricing models.
Proximity to emergency services is another interesting consideration. Blocks close to police and fire stations might receive lower insurance rates due to the perceived quicker response times. It appears that insurers see the potential reduction in accident severity as a mitigating factor, implying that the spatial relationship of public safety services influences their calculations.
It seems that parking availability also influences pricing. Blocks with limited parking options often have higher premiums because vehicles parked on streets face greater risk of damage or theft. Insurers likely recognize this and factor it into their pricing, adding to the complex interplay of factors at the block level.
Areas with robust alternative transportation systems—subways or ride-sharing services—may see lower insurance costs. It's logical that where people have options to substitute car use, insurers could see a lower risk of accidents. This demonstrates the broader effect of urban planning decisions on vehicle use and insurance pricing.
Finally, insurance rates in Manhattan show some seasonality. In summer, higher tourist numbers lead to heavier traffic, which in turn can increase the rate of accidents. This suggests a dynamic relationship between urban environments, traffic patterns, and the cost of insurance that insurance companies try to anticipate in their models.
How Recent Data Shows Location-Based Car Insurance Quotes Can Vary by 287% Within the Same City - Local Crime Data Links Insurance Costs to Neighborhood Safety Records
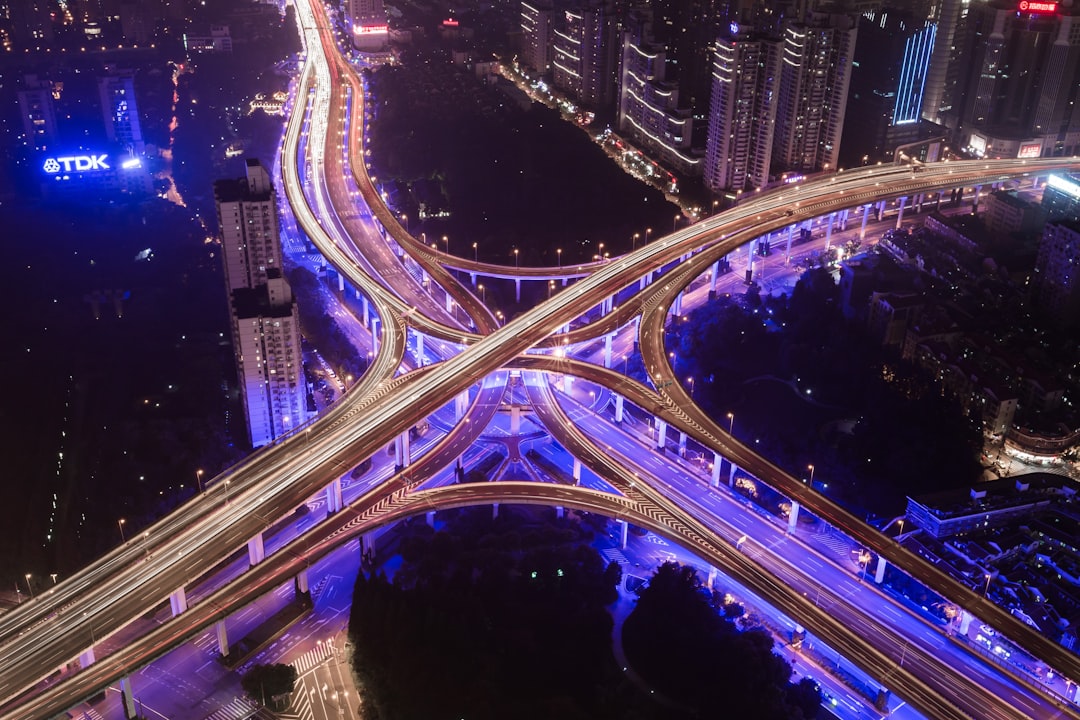
Insurance companies are increasingly using local crime data to assess risk and set premiums, particularly for auto and property insurance. This means that neighborhoods with higher crime rates, as documented by thousands of law enforcement agencies across the US, often face significantly higher insurance costs. Data analysis by insurers encompasses historical crime trends and incorporates current, real-time data to create a dynamic risk profile for each location. This can lead to dramatic disparities in insurance costs, with some neighborhoods experiencing premiums that are much higher than others within the same city, potentially as much as 287% higher. While this approach is understandable from the perspective of risk management, it raises concerns about equity. People living in neighborhoods with higher crime rates could be burdened with significantly higher insurance costs, even if their personal driving records are excellent. This practice highlights a complex issue: how can insurance pricing fairly and accurately reflect risk while also considering the broader socioeconomic factors that contribute to crime and inequality? The current reliance on location-based pricing, while seemingly practical for insurers, may unintentionally exacerbate existing socioeconomic disparities and inequalities within cities. It necessitates a deeper examination of the fairness and broader implications of relying so heavily on crime data to determine premiums.
Local crime data, particularly concerning violent crimes, is increasingly used by insurance companies to assess risk and set premiums. It's been found that neighborhoods with higher crime rates frequently experience significantly higher insurance costs. This correlation isn't just about the direct cost of crime-related claims; it also seems to impact the overall sense of safety in a community. In some cases, the perception of an area as unsafe can lead to further issues with crime, creating a challenging feedback loop.
However, the way insurers utilize location data can raise some questions. Their focus on pinpointing high-risk areas, primarily through geolocation, sometimes appears to ignore other factors, such as the broader socioeconomic conditions of those neighborhoods. This focus on a purely localized perspective could lead to skewed representations of risk.
For instance, the type of vehicles prevalent in a specific area also plays a role. Neighborhoods where particular car models are frequently targeted for theft often see insurance rates climb, even if the overall crime rate is relatively low. This raises questions about how broadly or narrowly insurers should define "risk."
This practice seems to suggest a type of behavioral economics phenomenon where insurers' actions can influence how people drive and behave on the roads. The fact that insurance costs increase in response to crime rates potentially leads to more cautious driving habits, although it can also impose a disproportionate burden on some residents.
Furthermore, the demographic composition of an area can sometimes lead to bias in insurance calculations. For instance, younger drivers in neighborhoods with a high car theft rate might face disproportionately higher premiums, a potential issue of equity. On the flip side, locations with strong community policing initiatives often see lower crime rates and correspondingly lower insurance costs. This suggests that crime prevention strategies can have a significant impact on the economic wellbeing of residents.
It's also interesting to observe that crime trends fluctuate with time. Car theft, for example, seems to increase during certain periods of the year, like the summer months. This implies that insurers have to constantly adapt their pricing models to account for both spatial and temporal patterns of risk. This is an ongoing dynamic process, not just a snapshot of risk.
Moreover, a high number of insurance claims from particular areas can create a feedback loop. Insurers, in response to numerous claims, often increase premiums not just for existing residents but also for future ones. This potentially perpetuates a cycle of higher insurance costs in those districts, which in turn can further exacerbate socioeconomic disparities.
Another element is how public transportation access can affect insurance costs. Areas with well-developed transit networks often experience lower insurance rates because people simply own and drive fewer cars. This lowers the overall risk of accidents and theft in the area.
It appears that neighborhoods with lower socioeconomic conditions can experience a combination of higher crime rates and elevated insurance costs. This double whammy can pose significant challenges for residents. Addressing this issue requires a holistic approach to urban planning and intervention. It’s a reminder that location-based pricing practices can have wide-reaching consequences that need to be thoughtfully considered and mitigated through various measures.
How Recent Data Shows Location-Based Car Insurance Quotes Can Vary by 287% Within the Same City - Weather Pattern Analysis Reveals Rate Differences Between City Areas
Recent examinations of weather patterns within cities have revealed that distinct weather characteristics can influence car insurance rates in localized areas. This finding suggests that insurance companies are increasingly using data on specific weather patterns, such as temperature variations and the frequency of severe weather events, to adjust insurance premiums. While this approach may be seen as a way to better assess risk, it also raises concerns about fairness and the potential for unintended consequences.
The analysis of weather data reveals how unique microclimates and weather patterns can contribute to different levels of risk in various parts of a city. It's possible that these patterns can lead to higher rates of accidents or certain types of vehicle damage, thus providing a justification for differential pricing. However, these localized models may not capture the full range of factors that influence driver behavior and risk. Further, the reliance on weather-related data for pricing raises questions about whether this approach exacerbates existing socioeconomic disparities in urban areas. It’s important to consider how insurance companies incorporate weather data into their models and whether this practice could lead to uneven burdens for residents depending on their geographic location within a city.
Examining the data, it's become clear that weather patterns aren't uniform across a city, leading to localized differences in driving conditions and accident rates. For example, if one neighborhood is more prone to fog or heavy rain than another, it's likely to see a higher frequency of accidents, and insurers may adjust premiums accordingly. This suggests a "microclimate effect" within cities that influences risk and subsequently, insurance pricing.
The use of advanced geospatial analytics allows insurers to examine data beyond just crime statistics. By mapping out factors like street lighting and weather conditions, it's possible to uncover subtle variations in risk even within a single city block. This approach generates a more detailed picture of local risk profiles, which may lead to a more nuanced approach to pricing, but also introduces questions about the equity of pricing based on factors outside of driving behavior.
Insurers aren't just relying on historical data anymore. They're increasingly incorporating real-time data into their models, such as the correlation between accident frequency and localized weather events. For example, an increase in collisions during a snowstorm might lead to more frequent premium adjustments. This trend towards "data-driven premium adjustments" introduces greater dynamism into the insurance market, though it raises questions regarding the balance between reactivity and potentially destabilizing rates based on short-term events.
There's growing evidence that weather patterns can indeed influence human behavior, including driving. For instance, drivers may adopt a more cautious approach during rain or snow, potentially leading to a decrease in accidents. However, this correlation between driving behavior and weather doesn't always translate into immediate changes in pricing. This suggests that while there are behavioral influences on safety related to weather, the correlation isn't perfectly predictable or immediately captured in pricing models.
We also see seasonal variations in traffic patterns and insurance rates. For example, winter months often experience a rise in accident claims, leading to an increase in insurance costs in certain neighborhoods. This "seasonal variability" emphasizes the complex interplay between weather, human behavior, and insurance pricing. It's not simply a matter of historical crime trends but also weather-related driving patterns.
Urbanization creates "urban heat islands," where certain areas experience higher temperatures compared to surrounding suburban or rural areas. This can lead to a higher incidence of engine overheating, which could potentially be factored into insurance premiums. In this way, we see how the localized urban environment influences risks that insurers may need to address with specific pricing adjustments.
The condition of localized infrastructure also appears to play a role. For example, poor drainage in certain areas can lead to localized flooding after heavy rain, potentially increasing both the risk of accidents and impacting the recovery rate of stolen vehicles. It's likely that insurers are incorporating these infrastructural elements into their risk models, and subsequent premium calculations.
Predictive modeling suggests that certain weather conditions lead to predictable spikes in accidents. By analyzing historical patterns surrounding similar weather incidents, insurers can potentially anticipate and adjust rates in advance. While this "behavioral predictability" enhances their risk management capacity, questions arise around the potential for over-reliance on models for pricing when other risk factors aren't fully integrated.
Interestingly, areas with certain weather patterns may see an increase in insurance fraud. The nature of fraudulent claims related to weather events necessitates more rigorous scrutiny and risk assessments by insurers. This raises the intriguing dynamic of insurance fraud linked to weather patterns and can result in higher premiums based on those assessments, adding an additional layer of complexity to the role weather plays in pricing.
Following severe weather events like hurricanes or major winter storms, the insurance market tends to respond by temporarily increasing premiums in affected areas. This demonstrates the very real and immediate influence of environmental disruptions on insurance dynamics. These "insurance market fluctuations" highlight the interconnectedness of extreme weather, insurance markets, and the economic wellbeing of urban communities.
How Recent Data Shows Location-Based Car Insurance Quotes Can Vary by 287% Within the Same City - Accident Frequency Maps Explain Cross Town Insurance Price Changes
Insurance companies are increasingly using detailed maps that pinpoint accident frequencies in various parts of a city to determine insurance rates. These maps reveal a clear correlation: areas with more accidents often face significantly higher insurance premiums. This reinforces the idea that location is a key factor in how insurers assess risk and ultimately set prices. However, this reliance on historical accident data raises questions about fairness. Residents in areas with high accident frequencies might be saddled with higher premiums, even if they are careful drivers with clean records. This raises concerns about equity and highlights a need to move beyond simply using accident rates as the sole factor in determining insurance costs. It suggests that a more balanced approach is needed, one that considers the social and economic aspects of neighborhoods along with accident data. How insurers utilize accident frequency data ultimately impacts the availability and affordability of car insurance for residents across a city, potentially creating disparities that are not tied to individual driving behavior.
It's becoming increasingly clear that car insurance pricing isn't just about a driver's history; it's also intricately tied to the specific environments where they live. Research shows that weather patterns, neighborhood infrastructure, and even microclimates can dramatically influence insurance premiums. For example, areas prone to fog or heavy rain may experience higher accident rates, leading to higher insurance costs. This connection between local geography and risk assessment is a fascinating area of study.
Interestingly, how weather influences driver behavior also appears to be a factor. We're finding that drivers generally take more precautions during adverse weather, potentially reducing accident risks. However, insurance companies aren't always quick to adjust premiums based on these changes in driving patterns, which raises questions about how well their models capture real-time behavior adjustments.
The quality of urban infrastructure can also make a difference. For example, neighborhoods with poor drainage are more likely to experience flooding after a storm, leading to a higher probability of car accidents and potentially impacting stolen vehicle recovery rates. This clearly highlights how urban design and infrastructure contribute to insurance costs, a finding that deserves more attention.
It's also become evident that insurance premiums fluctuate throughout the year. For example, winter months usually see an increase in accident-related claims, which naturally leads to higher insurance costs during those periods. This seasonal trend demonstrates that insurers are adjusting their pricing to match predictable environmental variations.
The urban environment's impact isn't limited to rainfall or snow. The phenomenon of urban heat islands, where localized temperatures rise due to urbanization, can also influence insurance pricing. Vehicle engine overheating issues become more common in these areas, which could factor into insurance rate calculations. It's an example of how urban design choices can have unanticipated financial effects on residents.
Insurers are increasingly relying on granular crime statistics to assess risk and set premiums. However, this approach can sometimes be overly simplistic, potentially ignoring broader social and economic conditions in certain areas. A sole focus on local crime rates may lead to unfair premium hikes for residents in lower-income communities, even if their individual driving records are excellent. This aspect of insurance pricing is a critical issue to explore from a societal perspective.
The rise of data-driven insurance models has brought real-time risk assessment into the field. Insurers are adjusting premiums based on the latest data, including localized weather patterns and other current factors. While this approach offers a level of responsiveness, it can also lead to greater price fluctuations and make insurance costs more unpredictable for consumers.
Some vehicle models are targeted more frequently for theft in specific neighborhoods, even in areas with relatively low overall crime rates. This leads to higher insurance costs for those who own those specific vehicles, emphasizing how specific features of a vehicle can affect insurance risk.
It's also noteworthy that a high number of insurance claims in an area can create a self-perpetuating cycle of increased premiums. Even if a neighborhood experiences social or economic improvements, insurance costs may stay elevated due to this "feedback loop."
Communities well-connected by public transport systems often see lower car ownership rates, leading to fewer accidents and insurance claims. This observation suggests that improved access to alternative transportation can lower insurance costs for residents in those areas. It's a compelling example of how urban planning decisions can have an impact on insurance pricing.
In essence, this research shows that location factors play a powerful role in shaping car insurance costs. Insurers are taking an increasingly nuanced approach to risk assessment, incorporating factors beyond driver history and expanding their understanding of urban environments. While this sophistication is understandable, it also highlights important social and economic implications that warrant further scrutiny and discussion.
More Posts from cashcache.co: