7 Strategic Investment Sectors Showing Growth Potential in Late 2024 From AI to Utilities
7 Strategic Investment Sectors Showing Growth Potential in Late 2024 From AI to Utilities - AI Hardware Manufacturing Set for 35% Growth Rate Through Q4 2024
The manufacturing sector focused on AI hardware is on track for substantial expansion, with forecasts suggesting a 35% growth rate by the end of 2024. This growth is fueled by the increasing need for AI semiconductors. These specialized chips are projected to contribute $71 billion to the global economy in 2024, a significant jump of 33% compared to the previous year. The rise of generative AI applications is a major catalyst, particularly in the demand for AI accelerators in servers. These accelerators alone are anticipated to reach $21 billion in value next year. However, the industry's ongoing growth will necessitate addressing issues surrounding data management and the potential risks associated with AI deployment. As AI capabilities become increasingly sophisticated, the manufacturing of the underlying hardware remains crucial, positioning the industry at the forefront of technological advancements.
The projected 35% growth in AI hardware manufacturing through the final quarter of 2024 is quite remarkable, especially given the already substantial investment and development in the field. It seems this growth is fueled by a combination of factors, including the push towards smaller and more powerful chips. These newer chip designs allow for more transistors within the same area, resulting in faster processing for the complex calculations that AI requires.
We're also seeing semiconductor companies increasingly invest in specialized hardware tailored to AI tasks. The development of ASICs, specifically crafted for neural network processing, seems to be a key aspect of this. This focus on dedicated hardware signifies a recognition that general-purpose chips may not be the best solution for many AI applications.
Furthermore, the rise of high-performance computing (HPC) is playing a crucial role. Industries across the spectrum are eager to utilize AI for complex data processing in real-time, pushing the demand for powerful machines able to handle such tasks. It's interesting to see how financial services, healthcare, and other sectors are becoming dependent on these capabilities.
Another aspect I find fascinating is the increasing importance of edge computing in this landscape. AI hardware is being incorporated directly into devices, instead of relying exclusively on centralized cloud systems. This addresses latency issues and allows for faster decision-making within operating environments. It'll be intriguing to see how this trend influences the development of AI within embedded systems.
Innovation in materials like gallium nitride (GaN) offers the potential to make even faster and more efficient chips compared to conventional silicon. This represents a key frontier for improving AI hardware performance. It remains to be seen how quickly these new materials are implemented, but the possibilities they present are considerable.
The competitiveness of this market is only going to increase. We're witnessing new players entering the AI hardware manufacturing space, often armed with disruptive technologies. This keeps the pressure on established players to push the boundaries of innovation to stay ahead. It's a rapidly evolving field.
And alongside innovation, the regulatory landscape is shifting. Scrutiny on AI hardware manufacturing processes is leading companies to adopt stricter quality control methods. It seems reasonable given that we're dealing with technology with a wide range of potential uses, some quite sensitive. We need to ensure that AI systems function reliably and safely.
There are intriguing possibilities with the emerging connection between AI hardware and quantum computing. It's still early days, but quantum computing's ability to handle complex calculations could fundamentally transform AI processing capabilities. While this technology may not be commercially available soon, it certainly has the potential to reshape the field down the line.
The growth of AI applications in critical areas like autonomous vehicles and robotics is also driving a surge in demand for advanced sensor technologies capable of interacting with AI hardware. This intertwining of tech areas suggests we may see rapid development in both sectors, influencing one another in complex ways.
Lastly, the investment in AI hardware isn't limited to traditional manufacturing methods. 3D printing is increasingly being used to fabricate complex components, demonstrating a shift towards customization and flexibility in hardware design. The possibility of quickly adapting hardware to specific applications could become a game-changer in some niche AI implementations.
7 Strategic Investment Sectors Showing Growth Potential in Late 2024 From AI to Utilities - Cloud Infrastructure Companies Expanding Data Centers by 40% for AI Workloads
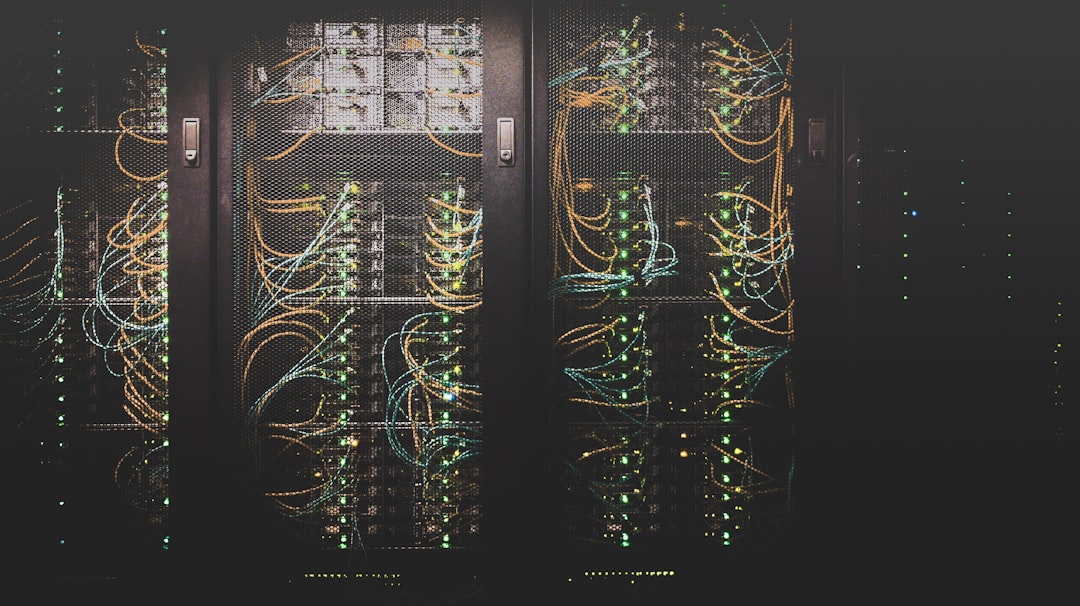
Cloud infrastructure companies are significantly expanding their data centers, with estimates suggesting a 40% increase in capacity to handle the surge in AI workloads. This expansion is being driven by the power-hungry nature of AI processing, particularly the specialized graphics processing units (GPUs) designed for these tasks. Traditional data center infrastructure struggles to keep pace with these advanced AI requirements. The need for these expanded capabilities is further highlighted by projections that data centers will double their energy consumption by 2030, potentially placing a strain on existing power grids. Major cloud providers are actively bolstering their infrastructure and adapting energy grids to accommodate these growing demands. The rise of AI, coupled with the burgeoning digital economy, appears to be the primary catalyst for this expansion. While the expansion is crucial for AI development, the long-term implications for energy consumption and sustainability deserve closer scrutiny as we move forward.
The surge in AI workloads is driving a significant expansion of cloud infrastructure, with data center companies planning to increase their capacity by roughly 40%. It's becoming increasingly clear that the current generation of AI models, especially those underpinning generative AI, are incredibly computationally intensive. To support this growth, we're seeing estimates that suggest millions of additional square feet of data center space will be needed by the end of 2025. This expansion isn't just about adding more servers; it's a response to a fundamental shift in how data is processed and moved.
One aspect of this expansion is a strong focus on low-latency connectivity, particularly as AI applications move beyond the cloud and into the realm of edge computing. This suggests a move towards a hybrid model, where processing power is distributed across multiple locations, rather than being centralized in a few massive data centers. This raises questions about how data centers will be integrated with local networks and the potential for increased complexity in infrastructure management.
Another key aspect of this infrastructure shift is the adoption of new technologies, especially in memory and cooling systems. We're seeing a wider use of high-bandwidth memory (HBM), which allows for much faster data transfer and access – crucial for the rapid processing AI demands. This is paired with a trend towards liquid cooling, which is more efficient than traditional air cooling, especially given the growing density of servers in these expanded facilities. I'm curious to see how these cooling methods influence the overall energy consumption of data centers, which is already a major concern.
Beyond hardware, we see software-defined networking (SDN) and network function virtualization (NFV) playing an increasingly important role in managing these complex environments. These technologies allow for more flexible allocation of network resources, improving efficiency and adaptability. It's interesting to note that AI itself is being used to optimize data center operations, with algorithms predicting cooling needs and performance bottlenecks. This kind of automation could help manage the rising complexity and costs of data center operations.
Furthermore, the expansion of data centers is creating an environment where interconnectedness and latency are paramount. The rise of multi-cloud strategies means data centers need to be able to communicate seamlessly, which requires a robust and adaptable network infrastructure. This also translates to a geographic diversification of data centers, with facilities being built closer to users to minimize delays in AI processing, creating new challenges and opportunities for the industry.
The increased reliance on AI is also driving up the need for storage capacity. As AI models become more complex and the data they consume grows, there's a corresponding demand for scalable storage solutions. These storage systems need to be able to grow dynamically alongside the increasing computational demands of AI applications.
It's also notable that the AI revolution is spurring innovation in chip manufacturing itself. The need for faster and more specialized chips, particularly GPUs capable of parallel processing, is driving new advancements in semiconductor fabrication. It'll be interesting to see how these developments impact both the cost and performance of AI systems in the coming years.
Overall, the expansion of data centers to support AI workloads paints a picture of a rapidly evolving landscape. We're seeing a blend of new technologies and strategies, from hardware improvements to software-defined networks, that are transforming the infrastructure that supports our digital world. The challenges are substantial, but so are the opportunities, suggesting a period of rapid development and innovation in this critical sector.
7 Strategic Investment Sectors Showing Growth Potential in Late 2024 From AI to Utilities - Renewable Energy Utilities Adding Smart Grid AI Systems for Peak Load Management
Renewable energy utilities are embracing smart grid AI systems to better manage peak energy demand. This is crucial as renewable energy sources, like solar and wind, can be unpredictable, leading to fluctuations in electricity generation. AI helps predict these fluctuations, allowing utilities to more effectively match energy supply with demand, preventing shortages and maximizing efficiency.
These AI-powered systems provide real-time monitoring of energy flow across the grid, improving the overall management of energy consumption and distribution. They can address challenges that come with renewable energy, such as the dependence on weather and geographical limitations. AI also aids in the maintenance and optimization of renewable energy equipment, extending the useful life of these systems and making them more reliable.
The complexity of power grids is rising, driven by the growing number of distributed renewable energy sources. This complexity makes forecasting essential, and AI excels at it. This reliance on AI is viewed as a vital component of advancing the use of renewable energy. As demand for energy is anticipated to rise in the coming years, this intelligent approach to grid management becomes more and more important for ensuring a stable and sustainable energy future.
Renewable energy sources, like solar and wind, are inherently variable, making it challenging for utilities to manage peak electricity demand. However, the integration of artificial intelligence (AI) within smart grid systems is changing the game. Government initiatives, like the US Department of Energy's $3 billion in grants for AI-powered smart grids, are pushing this trend forward. AI can improve grid management by leveraging sophisticated forecasting models. This means utilities can better anticipate and adjust to fluctuations in energy production from sources like solar panels, which are heavily dependent on weather.
Furthermore, AI-driven smart grids can monitor and control energy flows in real-time. By analyzing data from the Internet of Things (IoT) devices, they can optimize energy efficiency and improve grid resilience. This includes aspects like identifying and responding to outages more quickly. AI's ability to analyze massive datasets also helps with tasks like capacity planning and transmission studies, which are crucial for integrating new renewable energy sources into the existing power grid. It can also expedite federal permitting processes for new projects, reducing bottlenecks in renewable energy development.
AI is also crucial in addressing some of the inherent limitations of renewable energy. Variable generation, high upfront capital costs, and the need for effective energy storage solutions are all concerns. AI-powered optimization can help improve the operational efficiency of renewable energy systems, and therefore enhance their long-term viability. It's worth noting that the sheer complexity of the power grid, with its millions of interconnected sources and fluctuating demand, necessitates the use of AI for reliable forecasting and grid balancing.
The increased complexity of the modern power grid, with a mix of traditional and renewable generation, is driving the need for AI in this sector. It's increasingly difficult to predict energy demand and supply with accuracy. AI-driven models can help predict energy needs, optimize renewable energy production schedules, and potentially reduce the need for traditional, fossil fuel-powered peaking plants during times of high demand. Essentially, the role of AI in this sector is growing because it is becoming vital for ensuring the stability and reliability of the grid as we transition towards a greater reliance on renewable energy sources. This trend is also linked to future energy demand. With a growing global population, the pressure on energy supplies is increasing, and AI solutions for managing the complexities of renewable generation are seen as a vital component for meeting future needs.
While this is a promising development, it's important to consider the challenges. One concern is the potential for cybersecurity threats within these interconnected systems. The increased reliance on AI and the internet also means vulnerabilities that need careful consideration. Furthermore, the adoption of AI-driven systems within the existing regulatory framework requires careful consideration. How do we ensure that AI-driven grid optimization promotes fairness and equity in energy access for all consumers? These are important topics that will need further research and discussion as we continue to integrate AI in our energy infrastructure.
7 Strategic Investment Sectors Showing Growth Potential in Late 2024 From AI to Utilities - Healthcare Tech Firms Rolling Out AI Diagnostic Tools in 2500 US Hospitals
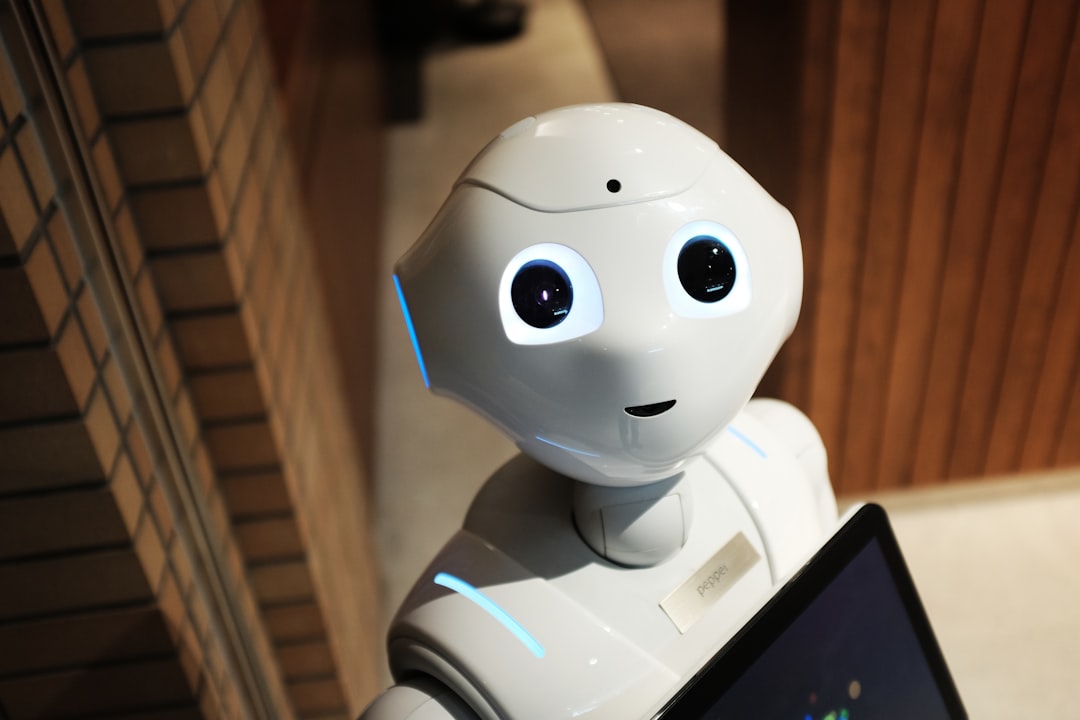
Artificial intelligence is making inroads into US hospitals, with healthcare technology companies deploying AI diagnostic tools in roughly 2,500 facilities nationwide. The goal is to improve the reliability and precision of medical diagnoses by using data analysis to minimize the inconsistencies inherent in traditional methods. This shift towards AI-driven diagnosis potentially leads to better patient outcomes and helps address the growing healthcare worker shortage. The global market for AI in healthcare is projected to grow substantially, highlighting a broader trend in the medical field toward the adoption of intelligent, automated solutions. While the potential of these AI tools is substantial, it is crucial to acknowledge and carefully consider the challenges and ethical questions that arise from integrating AI into such a critical and sensitive sector. As we progress through late 2024 and beyond, continued evaluation and understanding of these complexities will be essential to ensuring that AI's application in healthcare benefits both patients and medical professionals.
AI diagnostic tools are being rolled out in a substantial number of US hospitals, reaching 2,500 locations. It's quite a development, potentially leading to faster and more accurate diagnoses for a range of medical conditions. These tools use machine learning trained on extensive datasets to analyze medical images and patient information, sometimes with a speed and precision exceeding human capabilities. However, there's a notable degree of skepticism amongst healthcare professionals regarding the accuracy and ethical considerations of AI-driven diagnoses. Surveys suggest a significant portion of physicians express concerns about the reliability and potential biases of these systems, highlighting the importance of rigorous validation and transparent algorithms to foster wider acceptance.
It's interesting to see reports of reduced diagnostic errors in hospitals using these tools, with some showing a decrease of up to 15%. This is a significant potential improvement compared to traditional approaches, which are susceptible to human error. However, the introduction of AI has also prompted changes in the roles of some specialists. Radiologists, for instance, are finding their responsibilities shifting more towards oversight and interpretation, suggesting potential implications for job markets in the long run.
Naturally, ethical considerations are paramount. The algorithms underpinning these tools can inadvertently incorporate biases present in the data they're trained on. This has the potential to result in disparities in healthcare outcomes across different groups, so ongoing monitoring and refinement of these systems is essential to ensure equitable access and treatment.
Financially, the adoption of these tools shows some promise for healthcare cost reduction. Analyses indicate that AI diagnostic tools could lead to a decrease in overall costs, potentially by 10-20%, mostly through reduced readmissions and more efficient resource allocation. This has significant implications for healthcare budgets, especially in publicly funded systems.
Interestingly, some AI diagnostic tools utilize open-source datasets compiled from diverse populations, aiming to improve their accuracy across various demographic groups. This is a clever approach that also helps to minimize bias during the training phase. However, the growing reliance on AI has also introduced new cybersecurity risks. Hospitals employing these systems have reported an increase in cybersecurity incidents, such as data breaches and hacking attempts, rising by as much as 30%. This highlights the need for stringent data security measures to safeguard patient information.
The impact of AI isn't limited to diagnostics; it's also influencing patient management and treatment planning. AI can predict patient responses to specific treatments based on historical data, hinting at a future where healthcare is more personalized and tailored to individual needs. The potential for AI in healthcare is driving significant investment, with venture capital funding projected to potentially exceed $10 billion in the next few years. This influx of capital clearly reflects strong belief in the transformative potential of AI within healthcare, signaling continued innovation and development in this field.
7 Strategic Investment Sectors Showing Growth Potential in Late 2024 From AI to Utilities - Cybersecurity Providers Developing AI-Powered Threat Detection Networks
Cybersecurity is undergoing a transformation as providers increasingly leverage AI to create more sophisticated threat detection systems. We're seeing a significant rise in investment in AI-focused cybersecurity startups, especially those focused on securing applications and protecting sensitive data. The core of these new AI-driven tools is their ability to rapidly process and link together massive amounts of data, far faster than human analysts can, making threat detection faster. Yet, the success of these AI models relies heavily on the quality and reliability of the data they use for training, and their capacity to stay ahead of constantly changing cyberattacks. While the potential is huge, incorporating AI into cybersecurity also comes with its own set of challenges, particularly the need to create systems that are resilient to new forms of cyber threats. The need to keep security robust while AI evolves is a key factor in this burgeoning field.
The development of AI-powered threat detection networks by cybersecurity providers is gaining significant traction. It's notable that a substantial portion of cyberattacks exploit known vulnerabilities, suggesting that quicker threat detection and response are crucial for protecting organizational data and systems. This focus on faster response times is becoming increasingly important as the speed and scale of cyberattacks continue to increase.
AI algorithms are demonstrating a notable ability to learn and adapt. Some studies indicate that AI models can significantly improve their threat detection capabilities over time, potentially enhancing detection accuracy by 30% or more every few months. This ongoing improvement suggests that, when coupled with continuous updates, these systems could potentially maintain a lead against constantly evolving cyber threats. However, this advancement in cybersecurity also faces a counterpoint—the rise of AI-driven attacks. This symmetrical development suggests that hackers are using AI to improve their attacks in step with organizations' defensive AI enhancements, creating a continuous cycle of innovation and adaptation.
One of the most promising trends in this space is the application of unsupervised machine learning in threat detection. This approach allows systems to identify previously unseen threats, a fundamental change in how cybersecurity has traditionally been approached. Instead of solely reacting to known vulnerabilities, organizations are now in a better position to proactively address novel threats that haven't been previously encountered. This has significant implications for incident response time and the potential cost savings it can deliver.
Indeed, AI-powered systems are helping organizations reduce the time needed to respond to security incidents. In many cases, these systems have been shown to reduce response times by half or more, which can be critical in mitigating damage and reducing operational costs associated with security incidents. However, as with many sophisticated technologies, the use of AI in threat detection introduces new challenges. For instance, the complexity of some AI models makes it difficult for humans to understand how they arrive at a particular decision. This lack of transparency can be a barrier to wider adoption, especially in sectors where trust and accountability are critical.
Yet, AI continues to advance, particularly in the use of predictive analysis for cyber threats. Organizations are beginning to use AI systems to identify potential attack patterns based on historical data, allowing them to take preemptive steps to fortify their defenses. This proactive approach is fundamentally altering traditional cybersecurity strategies. In a related development, we are seeing a surge in the demand for security experts who can interpret the outputs of AI systems. While AI automation can reduce workload, the requirement to understand complex outputs in a nuanced way implies the need for skilled security professionals who can interpret AI analysis and translate it into effective defense actions.
At the same time, it's worth noting that even with AI advancements, human error continues to be a major factor in security breaches. It is reported that roughly 70% of breaches involve a human error, highlighting the importance of cybersecurity education and awareness among employees. It's a reminder that AI should be viewed as a complement to human expertise rather than a complete replacement.
Finally, we're entering an era where quantum computing could significantly impact the landscape of AI-powered threat detection. While still in its early stages, quantum computing's potential for breaking existing encryption methods suggests a potential need for significant reevaluation of cybersecurity protocols in the near future. As quantum technology progresses, it's a critical area for organizations to monitor and consider as they navigate the ever-evolving cybersecurity landscape.
7 Strategic Investment Sectors Showing Growth Potential in Late 2024 From AI to Utilities - Semiconductor Manufacturers Ramping Up AI Chip Production by 50%
Semiconductor companies are significantly increasing AI chip production, with estimates suggesting a 50% ramp-up to keep pace with growing demand. This is driven by the exploding AI chips market, which was worth roughly $53.7 billion in 2023 and is on track to surpass $71 billion this year, highlighting the crucial role these specialized chips play in powering AI applications. This trend suggests the overall semiconductor industry is poised for major growth, with some projecting global sales to hit a trillion dollars by 2030, propelled by the continuous innovation in AI.
However, the rapid expansion of AI chip production introduces complexities. The industry faces supply chain challenges, with chip lead times stretching significantly. Additionally, the use of AI in semiconductor manufacturing itself raises various ethical concerns, from data privacy to potential bias in automated processes. Companies across industries are incorporating AI into their operations, but navigating these issues is crucial as they strive to meet the burgeoning demand for the sophisticated hardware that underpins the AI revolution.
Semiconductor manufacturers are planning to significantly boost AI chip production by 50% in the coming months, a direct response to the escalating demand sparked by the rise of AI applications like machine learning and natural language processing. This surge in production underscores just how vital these chips are becoming across the tech landscape, with more industries looking to leverage AI capabilities in their operations.
The design of AI chips is also evolving, with a greater emphasis on application-specific integrated circuits (ASICs). These specialized chips are specifically crafted to optimize performance for particular AI tasks, such as neural network processing. It's a notable shift away from relying on general-purpose chips towards a model focused on maximizing efficiency and speed for AI workloads. This specialized approach reflects the increasing understanding that certain AI applications benefit greatly from custom-designed hardware.
Furthermore, the materials used in creating these chips are also undergoing a transformation. Alternative semiconductor materials, like gallium nitride (GaN) and silicon carbide (SiC), are being explored for their potential to create AI chips that can operate at higher frequencies and temperatures than traditional silicon. If successfully implemented, this could yield significant improvements in power efficiency and processing speed, benefiting both the performance and energy consumption of AI systems.
However, this increased production is also leading to a more competitive environment within the semiconductor industry. Nations are increasingly prioritizing domestic chip production capabilities, which is leading to a reshaping of the global semiconductor landscape. This situation introduces the need for enhanced collaboration among manufacturers, helping to streamline supply chains and minimize bottlenecks.
Beyond hardware, the push for AI necessitates improved data processing capabilities. By 2025, AI applications are projected to account for over 30% of global computing requirements, demonstrating a clear need for continued advancements in chip design and production. This surge in demand for processing power is shaping the trajectory of the chip industry as manufacturers strive to keep pace.
Intriguingly, the burgeoning field of quantum computing is starting to intersect with AI chip production. While still largely theoretical, AI algorithms may one day be deployed within quantum environments, which have the ability to solve certain problems at a speed far surpassing classical computers. This potential for future collaboration and synergy between these two advanced fields holds exciting possibilities.
We are also witnessing a noticeable increase in investment focused specifically on AI infrastructure. Many companies are dedicating a substantial portion of their technology budgets—about 25%—towards developing and integrating AI chips into their operations. This commitment highlights the belief that these technologies will provide strong returns on investment.
It's not all positive news, though. The semiconductor sector continues to grapple with significant chip shortages that could potentially hamper the projected growth in AI chip production. These supply chain issues, exacerbated by geopolitical tensions and pandemic-era disruptions, represent a significant challenge for manufacturers trying to meet demand.
Despite these challenges, AI adoption is spreading across a broader range of industries. Fields like agriculture and construction, traditionally not thought of as high-tech domains, are beginning to incorporate AI enabled by advanced semiconductor technology. This diversification of AI applications serves to expand the overall market for AI chips, furthering growth opportunities.
Lastly, the need for new chip designs is pushing the boundaries of fabrication techniques. Custom design methods, such as 3D chip stacking and modular designs, are being actively explored for their potential to not only improve performance but also increase manufacturing flexibility. This will enable companies to rapidly adapt to evolving technological demands as the AI landscape continues to evolve.
7 Strategic Investment Sectors Showing Growth Potential in Late 2024 From AI to Utilities - Green Energy Storage Companies Scaling Battery Production for Utility Integration
Green energy storage is experiencing a period of rapid expansion, with companies significantly increasing battery production to meet the growing demand for utility-scale energy storage solutions. This surge in production is a response to the increasing adoption of renewable energy sources, like solar and wind, which often generate electricity intermittently. Battery storage systems are crucial for stabilizing the grid by storing excess energy and releasing it when demand is high, ensuring a reliable power supply.
The growth in the sector is remarkable, with the global market for energy storage expected to reach a significant size in 2024. This momentum is further solidified by increasing investment, with billions of dollars funneled into the development of battery technologies. This is clearly indicating a widespread shift towards a more sustainable and resilient energy infrastructure.
While this scaling-up of production offers potential benefits, it also presents new challenges. Integrating large-scale battery storage into existing energy grids can be complex and requires careful planning and consideration. There are also potential issues surrounding the sustainability of the materials used in battery production, as well as the long-term impact on the environment from increased demand for resources. As the sector continues to expand, navigating these issues will be critical for ensuring the continued growth and viability of green energy storage solutions.
The landscape of utility-scale energy storage is undergoing a significant transformation, driven by the rapid advancements in battery technology. We're seeing a dramatic increase in battery storage installations, with the US alone adding 87 GW of capacity in 2023, a 90% leap from the previous year. This growth is mirrored globally, with the market adding 110 GWh of capacity, a staggering 149% year-over-year increase. This surge is attracting substantial investment, with the global energy storage sector seeing a 76% jump in funding, reaching $36 billion in 2023. It's remarkable that batteries are now the dominant form of energy storage, accounting for 90% of all new deployments since 2015. This strong emphasis on batteries is further supported by projections that anticipate the global market will reach 360 GWh in 2024, primarily powered by battery technology.
Interestingly, a significant portion of the investment and activity is focused on the industrial and utility sectors, highlighting the growing importance of energy storage for these industries. We even saw a noteworthy $225 million funding round for NineDot Holdings Inc., a sign of growing confidence in the industry. This growth isn't isolated; global energy investment is expected to exceed $3 trillion in 2024, with a significant portion—$2 trillion—allocated to clean energy technologies and infrastructure. It’s also telling that investment in renewables and storage now outpaces spending on traditional fossil fuels. This is a fascinating shift.
Battery energy storage systems (BESS) are becoming increasingly attractive for utilities due to their potential for reducing energy costs, particularly through techniques like peak shaving and integration with renewable sources. Estimates suggest that BESS can reduce energy costs by up to 80%. This is driven by the ability of these systems to store energy generated during periods of low demand (e.g., during off-peak hours) and release it during high-demand periods (e.g., peak hours), helping to balance energy production and consumption.
However, the long-term viability of BESS in utility grids requires careful consideration. While the cost of lithium-ion batteries has decreased significantly, it remains a critical factor. It will be interesting to see if further cost reductions are achievable and how effectively recycling methods can reduce the environmental impact of battery production and disposal. The lifespan and performance degradation of batteries are also key considerations for utility adoption. Long-term performance and the effectiveness of battery management systems to mitigate degradation will affect the economics and environmental impact of these technologies. Furthermore, the scalability of battery systems, integration with complex grid architectures, and ability to manage variable renewable energy inputs are critical factors influencing wider adoption.
There are many research and engineering challenges facing the development and integration of energy storage at scale. As the industry expands, these challenges will require innovative solutions that address the complexities of integrating BESS with existing grid infrastructures and effectively managing the operational and maintenance challenges associated with them. Overall, while the current trajectory is promising, there are considerable technical and economic challenges to overcome to ensure a seamless transition toward widespread utility-scale battery energy storage.
More Posts from cashcache.co: