How AI-Powered DTI Calculators Are Reshaping Financial Decision-Making in 2024
How AI-Powered DTI Calculators Are Reshaping Financial Decision-Making in 2024 - AI-driven DTI calculators enhance accuracy in financial forecasting
The integration of artificial intelligence into debt-to-income (DTI) calculators is transforming how financial forecasting is performed. These AI-powered tools leverage sophisticated machine learning techniques to sift through extensive data sets, uncovering hidden trends and irregularities that would likely be missed using traditional methods. This ability to uncover nuanced patterns leads to more accurate predictions and a deeper understanding of financial situations. The speed with which these AI-driven DTI calculators deliver insights is another key advantage. The automation they provide streamlines the forecasting process, enabling financial professionals to make decisions in real-time rather than waiting for days or weeks for static models to generate results. The shift towards automated, data-driven forecasting can lead to more effective risk management and strategic decision-making, impacting how organizations plan and manage their financial future. While this shift represents a significant advancement, it's important to acknowledge that relying solely on AI outputs without human oversight can have unintended consequences. The future of financial forecasting likely involves a blended approach where human expertise and AI collaboration work together to achieve optimal outcomes.
AI-powered DTI calculators are revolutionizing how we understand and forecast financial situations. While traditional DTI calculations often rely on simplified views of income and debt, AI algorithms can incorporate much more intricate details. For example, they can account for a wider variety of income sources and debt types, potentially boosting the accuracy of DTI calculations by a considerable margin, as indicated by recent research. Furthermore, these AI systems can continuously monitor spending habits, providing a more fluid picture of financial health compared to static calculations.
Some AI models are even incorporating natural language processing to analyze the context of user interactions. This ability to interpret conversations might reveal valuable insights about spending and income patterns often overlooked in conventional DTI methods. This, along with the capacity to analyze historical financial behavior and identify emerging trends and anomalies, helps predict future income stability and potential financial difficulties more precisely.
The integration of machine learning ensures that these calculators can adapt to evolving economic circumstances and individual financial habits. By constantly learning from new data, these AI models can improve their accuracy over time, making them more responsive to changes in the financial landscape.
Beyond personalized assessments, these advanced calculators can integrate external financial data like credit scores and market trends, giving users richer and more contextually relevant risk assessments. They can also simulate diverse financial scenarios – like job loss or unexpected expenses – that can significantly impact debt management strategies.
It's important to note that the improvements in AI security mean these systems can process sensitive financial information securely, while still providing thorough analysis and forecasting. The increasing accessibility of AI-driven DTI calculators through mobile applications represents a shift from older desktop-based systems, offering more convenience and flexibility for users to track their finances anytime and anywhere.
Despite the advantages, concerns remain about over-reliance on AI-powered tools. Simply relying on machine-generated insights could potentially overshadow the significance of personal financial literacy. A balanced approach, where AI enhances, but does not replace, informed decision-making remains crucial. This ensures that individuals continue to develop a sound understanding of their personal financial situation.
How AI-Powered DTI Calculators Are Reshaping Financial Decision-Making in 2024 - Generative AI shifts from experimentation to broader implementation
Generative AI, once largely confined to experimental settings, is now being integrated into mainstream operations across a range of industries. Fueled by rising interest and comfort with tools like ChatGPT and similar platforms, the shift marks a move from casual exploration to a more deliberate effort to incorporate generative AI into established workflows. While businesses are eager to adopt, they are also struggling to translate experimental enthusiasm into clear business strategies that demonstrate tangible benefits. There's a growing need to prove generative AI's worth and justify its implementation, a task that's proving difficult for many.
Despite the ongoing questions around ROI, early adopters seem poised to gain significant advantages. This suggests that generative AI is evolving into a pivotal technology for organizations that seek to optimize their operations and achieve sustainable growth. However, as the technology gains broader acceptance, ethical concerns will become increasingly important to address. As we move further into 2024 and beyond, striking a balance between realizing the potential benefits of generative AI and mitigating potential ethical pitfalls will be essential for its successful and responsible deployment.
The field of generative AI, once largely confined to experimental projects, is now transitioning into more widespread adoption across various organizations. This shift is fueled by a growing sense of curiosity and willingness among employees who've become familiar with tools like ChatGPT and Copilot. Businesses are moving beyond the trial-and-error stage, actively developing comprehensive strategies for incorporating generative AI into their core operations.
However, a key challenge remains: justifying the business value of implementing generative AI. While many employees are eager to experiment, some CIOs are struggling to demonstrate a clear return on investment for these implementations. This issue highlights the need for a more strategic approach to generative AI adoption, especially in industries with strict regulatory oversight like finance.
A recent Gartner report emphasizes the critical role of reusability in generative AI initiatives. Companies need to focus on creating strategies that maximize the reuse of models, data, and use cases. Doing so will contribute to sustained value creation and accelerate the time it takes to get projects from conception to practical application. Early adopters of generative AI technologies are expected to see a small but noticeable improvement in compound annual growth rate (CAGR), ranging from 0.5 to 0.7 percentage points by 2040. While later adopters will likely see some benefits, those gains are projected to be much smaller (between 0.1 and 0.3 percentage points).
McKinsey's 2023 annual survey reveals a surprisingly rapid adoption of generative AI tools across various industries. Less than a year after the launch of many of these tools, a third of surveyed organizations reported using generative AI in at least one of their business functions. This widespread adoption demonstrates the potential of these technologies, but also signals the need for careful consideration of their impact.
Generative AI's potential is being explored across many disciplines, leading to advancements in areas such as language processing, computer vision, and multimodal models. Technologies like GPT-3.5, GPT-4, and Pix2Seq represent major breakthroughs, but raise important questions about the ethical use of these powerful systems.
We are entering an era where companies are urged to see generative AI not as a threat, but as an opportunity to be pioneers. Just like in the early days of the internet, those who actively adapt and innovate with generative AI stand a greater chance of gaining competitive advantage. Those who wait too long risk being disrupted by competitors who are more adept at leveraging the technology. Ultimately, generative AI's potential to transform businesses is becoming undeniable. It is quickly being recognized as a key element in driving both efficiency and competitive advantage. We're only beginning to understand its full scope, and the coming years will likely reveal even more innovative applications of this technology.
How AI-Powered DTI Calculators Are Reshaping Financial Decision-Making in 2024 - Machine learning algorithms process large datasets for informed decisions
Machine learning algorithms are increasingly vital for processing the massive datasets that organizations now manage, allowing for more informed and timely decisions. These algorithms, unlike traditional methods reliant on human analysis alone, are adept at uncovering hidden patterns and trends within data. This ability to identify complex relationships improves their predictive capabilities over time, enabling more sophisticated decision-making, especially in situations requiring quick action. As AI evolves, these algorithms are enabling a shift away from simple predictive models toward more advanced optimization strategies. This has significant implications for fields like finance, where adaptive and efficient solutions are crucial for tackling dynamic challenges. It's crucial, however, to acknowledge the potential drawbacks of solely relying on machine-generated insights. There's a risk that such dependence could diminish the importance of human judgment and financial understanding, a balance that needs careful consideration as these systems become more prevalent.
Machine learning algorithms are adept at processing enormous datasets, encompassing billions of data points, which allows for a level of intricate analysis that would be impractical with traditional manual methods. This ability to scale is especially important for DTI calculators, which need to consider the wide range of financial situations across various demographics. It's fascinating how some machine learning models can achieve impressive predictive accuracy even with only a portion of the available data. This efficiency suggests the possibility of getting early financial insights without needing to crunch the entire dataset right from the start.
However, the sheer number of variables in some datasets can be a problem for machine learning, known as the "curse of dimensionality". Fortunately, there are advanced techniques, like dimensionality reduction, that help DTI calculators simplify complex financial models while preserving essential information. Interestingly, machine learning algorithms often use "ensemble methods," combining predictions from various models. This approach has been shown to noticeably boost prediction accuracy, a highly desirable feature for financial tools that assess risk.
Some more sophisticated machine learning algorithms, such as reinforcement learning, are capable of adapting in real-time based on a user's interactions with the calculator. This capability has the potential to generate personalized insights and advice that evolve as a user's financial situation changes. The ability of machine learning to spot outliers in large datasets can also provide early warnings about potential financial missteps. This proactive approach can be incredibly beneficial for users managing debt, as it helps them avoid common mistakes before they become bigger issues.
The question of how transparent machine learning models are is becoming increasingly important. Some approaches, like decision trees, provide a degree of clarity about how the algorithms make decisions, which can help build trust with users who might be hesitant to rely on AI systems that appear like black boxes. Financial data often has a time-based component, and machine learning models, particularly recurrent neural networks, are specifically designed to analyze this time-series data. This allows them to forecast trends and shifts in DTI calculations over time.
We are also seeing exciting new developments like causal inference within machine learning, which can allow DTI calculators to investigate not just correlations but also the underlying causes of financial behavior. This deeper understanding could lead to more insightful guidance tailored to each user's circumstances. Many machine learning algorithms can continuously incorporate new financial data, learning in real-time from fresh inputs. This means DTI calculators can stay relevant and accurate, adapting to shifts in the economic landscape and each user's individual circumstances without needing constant retraining. It's a constant process of learning and refinement, driven by the ever-changing financial world we live in.
How AI-Powered DTI Calculators Are Reshaping Financial Decision-Making in 2024 - Predictive analytics transforms risk assessments in finance
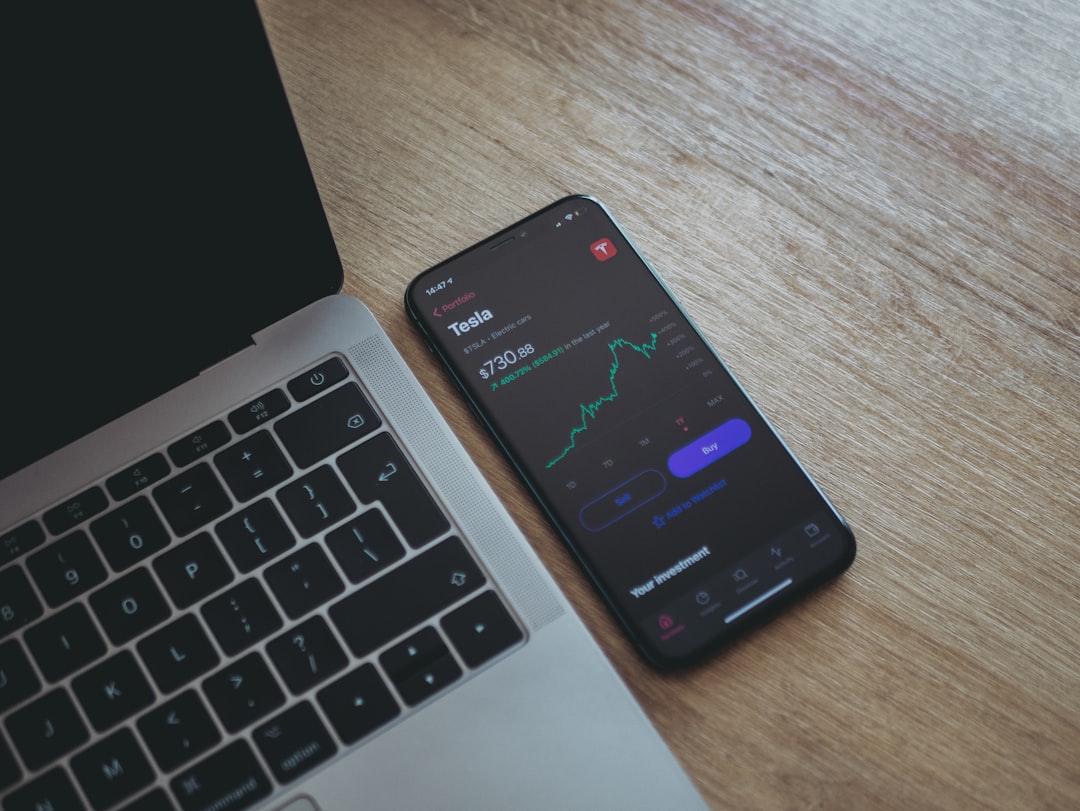
Predictive analytics is transforming how financial institutions assess risk, using sophisticated machine learning and vast datasets to gain a deeper understanding of potential threats. This change allows for more precise forecasting of market trends, customer behavior, and emerging risks, leading to improved decision-making and potentially, better profitability. By automating tasks and streamlining risk management processes, predictive analytics helps enhance operational efficiency. This includes applications in detecting fraudulent activities, assessing creditworthiness, and ensuring compliance with regulations. While these advancements are promising, hurdles remain. Concerns about data privacy, the potential for biases in algorithms, and the need for greater transparency in how these systems operate are essential factors to consider. The move to predictive analytics represents a clear departure from older, more static risk assessment methods, marking a shift towards more accurate and adaptable financial risk management.
The application of predictive analytics is fundamentally changing how risk is assessed in finance. It's fascinating how these techniques, built on machine learning and fueled by vast datasets, can deliver a level of precision in forecasting that traditional methods simply couldn't achieve. We're seeing improvements in risk assessment accuracy, with some studies indicating increases of up to 25%. This enhanced precision is valuable for making decisions around lending and investments, reducing the uncertainty that's inherent in financial markets.
Beyond just crunching numbers, predictive analytics can also tap into the realm of human behavior. Analyzing social media activity, spending patterns, and other signals, it becomes possible to get a sense of a person's future financial health, which is intriguing from a research perspective. It's like having a crystal ball, but based on rigorous statistical models instead of magic. Furthermore, sophisticated predictive models can quickly simulate a range of economic scenarios, offering real-time insights into how risk levels might change. This ability to rapidly understand potential impacts on a portfolio or a loan book empowers financial professionals to be more agile and proactive in their decision-making.
The continuous learning capabilities of these models are remarkable. The more data they encounter, the better they get at fine-tuning predictions and adapting to evolving market conditions. This is a very powerful aspect, as it means the system isn't static but rather continuously improves its relevance over time. It's not without challenges, though. The sheer volume of data can be overwhelming, leading to the "curse of dimensionality" where things become overly complex. But researchers are addressing this, leveraging techniques like dimensionality reduction to effectively summarize information without losing key details. This is vital in making risk assessment practical.
One of the more interesting applications is anomaly detection. Predictive models can spot unusual transactions or behavior, acting as an early warning system for potential fraud or financial instability. This aspect is crucial for protecting financial institutions and consumers alike. While these advancements offer incredible potential, the pursuit of cost efficiency is another major factor. Some firms report cost reductions of up to 30% by automating tasks and reports that used to require significant manual effort.
Moving beyond just risk, we're also seeing applications in market trend forecasting. Predictive models have achieved impressive accuracy in certain areas like stock market predictions, reaching levels of 85% in some cases. But I think one of the most exciting areas is the recent emphasis on causal inference. Instead of simply showing correlations, we're moving toward understanding the causal relationships between different financial factors. This is fundamental for offering advice on how to improve financial outcomes rather than just predicting what might happen. And it's not just a one-size-fits-all approach. Predictive analytics allows for highly personalized risk assessments, by incorporating an individual's past actions, preferences, and real-time behavior. It truly is moving towards a future where finance is increasingly tailored to each person's unique circumstances.
Of course, like any powerful tool, there are concerns about biases and transparency. As we further integrate these techniques into financial systems, it will be important to carefully consider how to mitigate potential pitfalls and maintain a balanced approach. Nevertheless, I see predictive analytics as a key element driving change in the financial world. It has the potential to reshape the way we make decisions, reduce risk, and even improve financial well-being. It's an incredibly exciting field to be watching as it continues to evolve and reshape the landscape of finance.
How AI-Powered DTI Calculators Are Reshaping Financial Decision-Making in 2024 - AI chatbots revolutionize customer interaction in banking
AI chatbots are transforming how customers interact with banks, offering a level of efficiency that traditional methods often fail to achieve. These intelligent systems not only streamline customer service but also provide immediate answers to questions, improving the overall experience and potentially fostering greater customer loyalty. Through natural language processing and machine learning, AI chatbots can adapt to individual customer needs, creating personalized interactions. This customization could play a key role in client retention and potentially lead to better financial outcomes. However, while AI chatbots bring powerful capabilities, there's a risk that over-reliance on automated solutions could overshadow the importance of human interaction and personal financial understanding. The correct approach needs to blend automation with the right kind of human oversight. As 2024 progresses, the integration of these AI-driven tools is likely to continue reshaping customer interactions within the banking sector, potentially having a profound influence on the entire customer journey.
AI chatbots are increasingly becoming a standard feature in the banking landscape, fundamentally changing how banks interact with their customers. Their ability to handle numerous conversations concurrently means banks can offer near-instantaneous support and information around the clock, a level of service that would be impossible for human agents to match on a large scale. The continuous advancements in natural language processing are also noteworthy. Chatbots are getting better at deciphering complex requests and responding in a way that feels more conversational and personalized, leading to higher customer satisfaction.
Interestingly, banks that have adopted these AI systems report cost reductions of up to 30%. They're able to automate a significant portion of basic queries, freeing up human staff to address more complex issues that necessitate a nuanced understanding. This 24/7 availability is another crucial element, as it extends assistance beyond standard business hours. This continuous access can potentially improve customer retention rates, especially for those needing immediate support.
Some AI chatbots are even integrated with advanced predictive analytics, enabling real-time detection of unusual transaction patterns. This proactive fraud prevention feature not only safeguards banks but also offers a crucial layer of security to their customers. Beyond operational efficiency, these AI interactions produce a wealth of data, providing insights into customer behavior and preferences. Banks can then utilize this understanding to tailor marketing strategies and product development efforts, making services more relevant to individual customer needs.
Furthermore, many chatbots can effortlessly integrate with various platforms like social media, mobile apps, and bank websites, offering a unified and streamlined customer service experience, regardless of where the interaction originates. Through these chatbots, customers can also accomplish tasks like checking balances, making transfers, or applying for loans without navigating overly intricate systems, fostering a greater sense of independence and satisfaction. This also makes interactions much more efficient, reducing the frustration that customers sometimes encounter when navigating traditional banking systems.
These AI systems can play a significant role during financial crises, acting as an automated conduit for critical information and advice to a broad customer base. This capability can potentially help calm concerns and manage anxieties during periods of uncertainty, demonstrating a proactive stance from banks to their clients.
However, there are limitations. Despite significant advancements, chatbots still struggle with mirroring genuine human empathy in their responses. This can lead to some challenges in situations where customers are dealing with emotionally charged scenarios. It highlights the importance of a combined approach where human agents are available to step in when needed, maintaining a balance between efficiency and human connection. As the field continues to develop, we're bound to see AI chatbots evolve further, offering more refined and helpful interactions in the banking sphere, though the need for a balanced approach with human involvement seems likely to remain.
How AI-Powered DTI Calculators Are Reshaping Financial Decision-Making in 2024 - AI models contribute to precision in financial decision-making processes
AI models are revolutionizing financial decision-making by leveraging advanced algorithms and machine learning to analyze vast amounts of data. This heightened analytical capability allows for more precise assessments of factors like credit risk and individual financial stability, directly impacting areas like loan approvals and risk management strategies. The capacity of these AI models to continuously learn and adjust to shifting financial conditions is particularly valuable, resulting in more accurate predictions over time. However, achieving the full potential of AI in finance necessitates a careful approach. Maintaining ethical considerations and mitigating potential biases within AI models is crucial to ensure responsible application. As 2024 unfolds, the integration of AI into financial operations is poised to transform decision-making processes, but it also presents challenges that require careful attention and a balance between AI insights and human judgment.
AI models are increasingly crucial in refining financial decision-making processes by leveraging sophisticated algorithms and machine learning techniques to sift through massive datasets. They can process enormous amounts of data, revealing hidden patterns and relationships that would be missed by traditional methods, leading to greater precision in financial assessments. For example, these models can tap into decades of historical financial data, uncovering long-term trends and seasonal patterns that are helpful in understanding current DTI (Debt-to-Income) situations and making future projections.
Further, some AI models are designed to dynamically adjust their algorithms based on real-time economic indicators and user interactions. This means they can quickly adapt to shifts in the financial landscape, making financial forecasting more responsive. Beyond simply identifying correlations, advanced techniques now allow these models to investigate causal relationships in financial behavior. This deeper understanding of "why" specific financial patterns occur can inform more targeted and effective strategies.
Research suggests that implementing machine learning for DTI calculations can substantially improve prediction accuracy by as much as 25%. This boost in precision is particularly useful for assessing risk and making informed decisions, especially in fluctuating markets. Additionally, some AI systems include mechanisms to analyze their own outputs for potential biases. These self-auditing capabilities are crucial for promoting fairness in financial assessments and are important for upholding user trust and meeting regulatory standards.
AI models are also proficient at identifying unusual patterns or outliers in financial behavior. This anomaly detection function can serve as an early warning system for fraud or mismanagement, providing a significant enhancement to risk management efforts. The evolution of AI in finance is not isolated. It's drawing upon insights from other fields like healthcare and retail. Techniques for analyzing customer behaviors in these industries are now being adopted in financial decision-making, leading to innovative improvements in accuracy.
Moreover, there's a growing integration of data from the Internet of Things (IoT), like smart home technology and wearables, into financial models. This integration creates a more complete picture of an individual's financial health by incorporating lifestyle factors that influence spending and saving behaviors. Furthermore, AI models can generate a wide range of financial scenarios based on different inputs, enabling users to visualize potential outcomes of various financial decisions. This dynamic simulation capability fosters more informed and confident decision-making, especially within complex financial environments. While the potential of AI in finance is immense, researchers must also remain vigilant about responsible development and ethical considerations to ensure these systems benefit all stakeholders.
More Posts from cashcache.co: